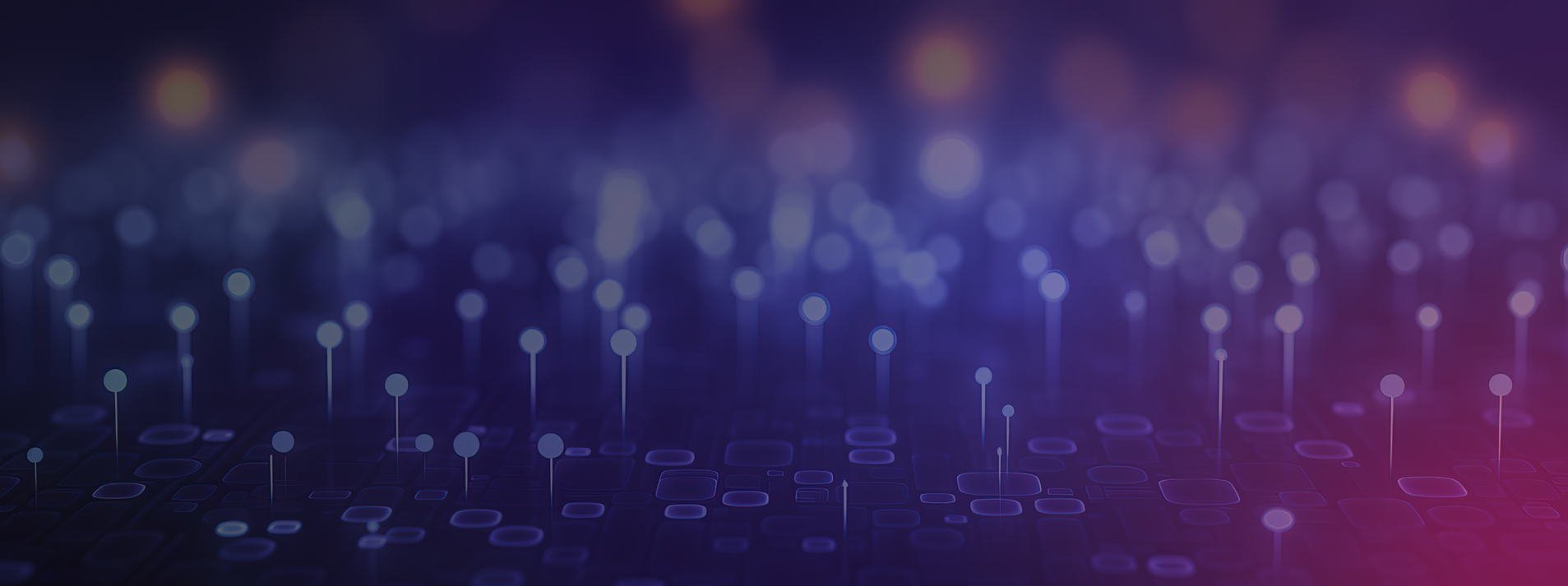
Preparing Your Business for AI Adoption – 6 things to consider
An exploration of risks and opportunities for businesses when adopting AI
Share this
Publish date: 10.04.25
The integration of artificial intelligence into modern business processes represents both a technological evolution and a paradigm shift. While the foundational concepts behind AI—particularly machine learning—have been embedded in enterprise and consumer systems for years, the current wave of innovation is redefining what’s possible, and doing so at a pace that challenges traditional governance, risk, and compliance frameworks and privacy laws.
AI’s Ubiquity and Acceleration
AI in the workplace or in our daily lives isn’t a new concept. For well over a decade now, we’ve seen machine learning quietly powering a host of features automated we now take for granted—predictive text on smartphones, real-time transcription during video calls, intelligent email summarisation, automatic language translation, personalised marketing, and content recommendations. These implementations, though valuable to businesses and far-reaching, were largely narrow in scope and limited to specific tasks with a very clearly defined structure.
However, the release of ChatGPT in November 2022 marked a clear inflection point in AI development and visibility within the business world, introducing generative AI – for free – to millions workers world-wide.
Generative AI has not only captured public imagination but has also accelerated board-level interest in AI deployment. The technology is maturing and now demonstrates capabilities that go beyond task automation—delivering content which looks and sounds increasingly human-like and enabling a host of new business functionality. A lot of knowledge work, once thought immune to automation, is now squarely within the reach of generative AI systems’ capabilities.
This rapid advancement has led to high-profile discussions among global regulators, policymakers, and corporate leaders as well as the larger public. While the opportunities seem almost limitless, the risks—especially in cybersecurity and data governance—are equally profound.
With this in mind, what are the key points of interest for your business when considering AI adoption across your organisation?
1. Security Implications: The Double-Edged Sword of AI
AI systems offer compelling efficiency gains and new capabilities, but they also widen the threat surface. One of the most significant developments is the democratisation of hacking. Generative AI tools can now enable cybercriminals without advanced skills to craft sophisticated attacks—from persuasive phishing emails to polymorphic malware—without requiring traditional technical expertise. This development drastically lowers the barrier to entry for malicious actors.
Furthermore, the sophistication of AI-generated threats makes them harder to detect with legacy security systems. These threats can easily evade signature-based defences, requiring enterprises to adopt more adaptive and AI-driven threat detection mechanisms. Internal risks are equally concerning: employees deploying AI tools without proper oversight may inadvertently leak sensitive data, misconfigure permissions, or interact with models in ways that open up attack vectors.
2. Mitigation Strategies: Building a Resilient AI Deployment Framework
To manage these evolving risks, companies must take a structured, proactive approach. Strong data governance and access control are foundational. Since AI systems often require extensive datasets to perform effectively, strict access controls must be enforced. Organisations should restrict access to sensitive information, audit training and inference data for compliance with privacy regulations, and avoid uploading confidential or personal data to public or third-party AI systems without robust safeguards in place.
Operational constraints also need to be clearly defined. For example, a customer-facing chatbot trained on general-purpose data might inadvertently recommend a competitor’s product or service, potentially damaging client relationships and brand integrity. AI systems should be deployed within clearly scoped domains, and outputs should be monitored for accuracy, relevance, and risk.
Compliance and ethical oversight must extend beyond AI-specific regulations. AI centralises data in ways that increase the blast radius of a breach. If compromised, these systems can leak sensitive customer data, intellectual property, or operational insights. Inadvertent data exposure or model misuse can also lead to violations of privacy laws like GDPR or CCPA, and trigger both legal and reputational consequences. Intellectual property is another concern, especially when AI models are trained on proprietary data or open datasets that may contain protected content. This introduces potential legal ambiguity around content ownership and usage rights.
3. Bias, Transparency, and Fairness
AI systems, especially those trained on large and uncurated datasets, are prone to replicating and even amplifying existing societal biases. These algorithmic biases can manifest in critical processes like hiring, lending, or customer support—leading to unfair and discriminatory outcomes. The consequences extend beyond reputation; biased AI decisions can violate anti-discrimination laws and result in regulatory scrutiny or litigation.
Adding to this concern is the inherent opacity of many AI models. The so-called “black box” nature of deep learning systems makes it difficult to explain how certain decisions are made. This lack of transparency can erode trust and make it difficult to enforce accountability or ensure fairness. Organisations deploying AI should invest in explainable AI techniques, implement regular bias audits, and keep human oversight in the loop—particularly in high-impact decision areas.
4. Economic and Workforce Impacts
While AI promises significant productivity gains, it also brings the potential for widespread workforce disruption. As AI automates an increasing range of tasks, job displacement is a real concern across industries—from manufacturing to white-collar sectors such as legal and finance. Without mitigation, this could lead to social pushback and internal resistance to adoption.
To navigate this transition, businesses must make deliberate investments in workforce development. The focus should be on upskilling and reskilling existing employees in areas such as data literacy, AI model oversight, and prompt engineering. This not only reduces displacement risk but also ensures that human workers remain essential participants in AI-augmented processes. Companies that fail to adapt their talent strategies risk being left behind—not by technology, but by a lack of capability to use it effectively.
5. Operational Risks and Over-Reliance
AI systems are not infallible. They can make incorrect or misleading predictions with high confidence, often referred to as hallucinations. These errors, while sometimes harmless in casual use, can have serious consequences in business settings—especially when used in areas like financial forecasting, legal review, or customer service automation.
The risk of poor decision-making is compounded when organisations become overly reliant on AI without adequate human supervision. A fully automated pipeline that acts on flawed AI output may make decisions that harm clients, breach compliance standards, or result in financial loss. Complicating matters further, AI systems don’t fit neatly into traditional accountability models. When an AI-driven action causes harm, determining responsibility—whether with the developer, the deployer, or the vendor—remains a grey area. Establishing clear governance frameworks for AI accountability is becoming critical.
6. Environmental Considerations
The environmental cost of AI, often overlooked in strategic planning, is increasingly important. Training large AI models and running inference workloads at scale require enormous computational power. According to the European Parliament, digital infrastructure consumed 7% of the world’s electricity in 2021, with projections suggesting this could rise to 13% by 2030. AI workloads, especially those hosted on cloud platforms with general-purpose hardware, contribute significantly to this consumption.
Companies should incorporate sustainability into their AI procurement and deployment decisions. This includes assessing vendors based on their renewable energy commitments, data centre efficiency, and environmental reporting. Encouragingly, many major cloud providers are aggressively pursuing net-zero strategies and have made significant investments in green energy. For certain workloads, deploying AI inference to edge devices or specialised AI PCs can reduce the energy burden dramatically. These localised systems not only consume less power but also offer latency and privacy advantages.
Conclusion: Strategic AI Adoption Requires Dual Focus
AI is undeniably a force multiplier for innovation, but without intentional design and governance, it can quickly become a liability. Businesses that will thrive in the AI era are those that embed security, ethics, and operational resilience into their AI strategies from the ground up. This means developing systems with built-in privacy and safety mechanisms, aligning deployment with regulatory and environmental standards, and fostering an adaptive workforce prepared for rapid technological evolution.
Ultimately, the goal is not just to use AI—but to use it responsibly, sustainably, and securely. Innovation and risk management are not opposing forces; in the age of AI, they must evolve together.